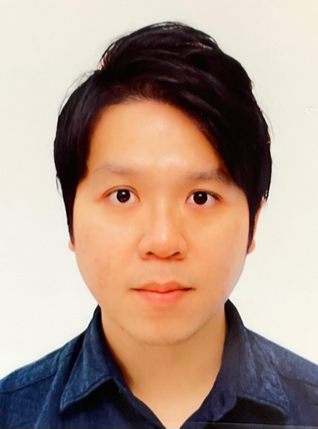
Research Theme
Growing Memory Neural Model for Continual Learning of Intelligent Agents
Keywords
lifelong learning, self-organizing, incremental learning, topological network
Introduction
The general success criterion for an artificial intelligence system is its ability to mimic human brain learning. Throughout a lifetime, the human brain is capable of continual learning. The acquired information is kept, augmented, fine-tuned, and utilized to complete new tasks in the future. At the moment, machine learning models perform well when given precisely structured, balanced, and homogenized data. However, when several jobs with incremental data are provided, the performance of the majority of these models suffers. Inspired by the Complementary Learning Systems (CLS) theory in neuroscience, episodic-semantic memory-based frameworks have received much attention and research. On the other hand, conventional methods are needed to perform data batch normalization and are sensitive to vigilance hyperparameters across different datasets. This paper proposes a Robust Growing Memory Network (RGMN) that continuously learns incoming data without normalization and is unlikely to be affected by the vigilance hyperparameter. The RGMN is a self-organizing topological network that models human episodic memory, and its network size can grow and shrink in response to data. The long-term memory buffer retains the largest and smallest data values that will use for learning. To evaluate the performance of the proposed method, we conducted comparative experiments on real-world datasets, and the results showed that the proposed method outperforms existing memory-based baseline frameworks in terms of accuracy.
Proposed Method
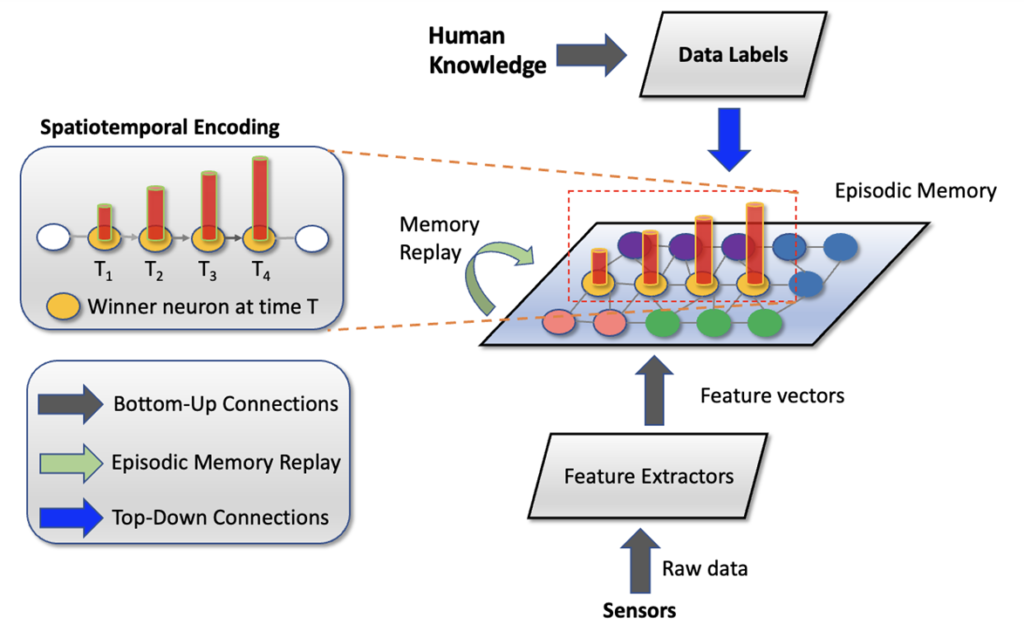
Research Findings
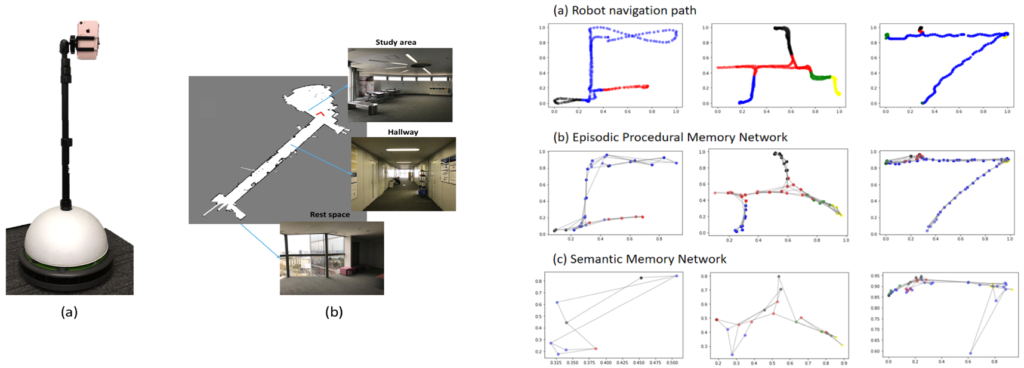